Abstract
Quantifying activity from acceleration signals during sleep is a key step in assessing sleep quality. However, the detailed methodology underlying the derivation of many actigraphy metrics typically is a black box for users of commercial actigraphy devices. To fully understand the strengths/limitations of these devices and to elaborate the technical factors that underlie them, we conducted a series of studies to examine the methodological algorithms for two widely used actigraph devices, Motionlogger Sleep Watch (AMI, NY) and Actiwatch-2 (Phillips-Respironics, Bend, Oregon). This document provides a conceptual introduction to the methods of quantifying activity and then discusses the motivation for conducting the series of studies.
1. Two Methods of Quantifying Activity
Two commonly used modes for deriving activity are proportional integrating mode (PIM) and zero crossing mode (ZCM).
PIM involves sampling the accelerometry output signal at a high rate, then calculating the area under the curve [1-2]. For example, Figures 1a and 1b illustrate the acceleration signal of periodic hand shaking with different amplitudes. There are 10 hand shakes over a 5-second span with amplitude of approximately 200 (Figure 1a) and 100 mG (Figure 1b). The cumulative area under the curves are 600 and 350 mG • s, respectively. PIM is usually considered a measure for activity magnitude or motion vigor.
On the other hand, the zero-crossing (ZC) count is the number of times the signal crosses zero (or near zero), labeled as red circles in Figure 1. Although the amplitude in Figure 1a is greater than that in Figure 1b, the frequency of the movements and the ZC counts are similar. Thus, ZCM mainly reflects movement frequency, while PIM reflects movement amplitude.
.webp)
2. Comparison between PIM and ZCM in Sleep Analysis
Motionlogger Sleep Watch (AMI, NY) and Actiwatch-2 (Phillips-Respironics, Bend, Oregon) are the two most commonly used actigraph devices for sleep analysis [1,3]. Motionlogger records activity based on both ZCM and PIM, and Actiwatch-2 is based solely on PIM mode. According to the Motionlogger User’s Guide, ZCM is the suggested mode due to its high accuracy [4]. However, some studies suggest that the PIM leads to better results [5-6].
For between-device comparisons, Meltzer et al. found that both the Motionlogger ZCM and Actiwatch-2 PIM provided good sensitivity to detect sleep (89% to 97%) and overall accuracy of sleep/wake identification (87% to 90%) when comparing to the gold-standard polysomnography (PSG). However, the specificity for detecting wake was poorer (54% to 77%). Furthermore, the two brands showed poor agreement on the total sleep time, wake after sleep onset, and sleep efficiency [7].
Fekedulegn et al. pointed out that the mode selection should depend on several factors, including age, gender, underlying disease of the study population, and the research question. Consequently, mode selection and some parameter determinations remain unsettled and are expected to be highly population-specific and personalized [1,8].
3. Current Limitations and Goal of Our Series Studies
The users can easily obtain detailed sleep reports through the available friendly interfaces and simple commercial softwares [4,9]. However, the algorithm for deriving sleep measures from raw acceleration signal is rather complicated with many layers for adjusting various parameters.
We conducted a series of studies to explore how these commercially available devices derived sleep parameters from the processed acceleration signals. We also explored how certain hardware and software variables influence the final sleep reports. Factors considered in these studies include the type of accelerometer sensor, choice of acceleration processing modes, bandpass filter settings, threshold for slight movement, among others.
Our aims for these studies are (1) to provide full transparency regarding the processing methodologies for actigraphy analysis and (2) to afford wider options for mode selection and parameter adjustments to effectively meet the customized/personalized needs of different research.
4. References
[1] D. Fekedulegn, M. E. Andrew, M. Shi, J. M. Violanti, S. Knox, and K. E. Innes, "Actigraphy-based assessment of sleep parameters," Annals of Work Exposures and Health, vol. 64, no. 4, pp. 350-367, 2020.
[2] S. Ancoli-Israel, R. Cole, C. Alessi, M. Chambers, W. Moorcroft, and C. P. Pollak, "The role of actigraphy in the study of sleep and circadian rhythms," Sleep, vol. 26, no. 3, pp. 342-392, 2003.
[3] L. J. Meltzer, H. E. Montgomery-Downs, S. P. Insana, and C. M. Walsh, "Use of actigraphy for assessment in pediatric sleep research," Sleep medicine reviews, vol. 16, no. 5, pp. 463-475, 2012.
[4] Ambulatory Monitoring, Inc. Motionlogger user guide.
[5] T. Blackwell et al., "Comparison of sleep parameters from actigraphy and polysomnography in older women: the SOF study," Sleep, vol. 31, no. 2, pp. 283-291, 2008.
[6] T. Blackwell, S. Ancoli-Israel, S. Redline, K. L. Stone, and O. F. i. M. S. Group, "Factors that may influence the classification of sleep-wake by wrist actigraphy: the MrOS Sleep Study," Journal of clinical sleep medicine, 2011.
[7] L. J. Meltzer, C. M. Walsh, J. Traylor, and A. M. Westin, "Direct comparison of two new actigraphs and polysomnography in children and adolescents," Sleep, vol. 35, no. 1, pp. 159-166, 2012.
[8] M. Rowe et al., "Actigraphy in older adults: comparison of means and variability of three different aggregates of measurement," Behavioral sleep medicine, vol. 6, no. 2, pp. 127-145, 2008.
[9] Respironics (2018). Actiwatch Communication and Sleep Analysis Software. Instruction manual.
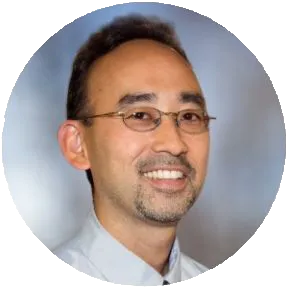
Dr. Ahn is an internal medicine physician with a background in physics/engineering and physiological signal analyses. He is the Chief Medical Officer at Labfront and an Assistant Professor in Medicine & Radiology at Harvard Medical School. Dr. Ahn is passionate about democratizing health sciences and exploring health from an anti-disciplinary perspective.
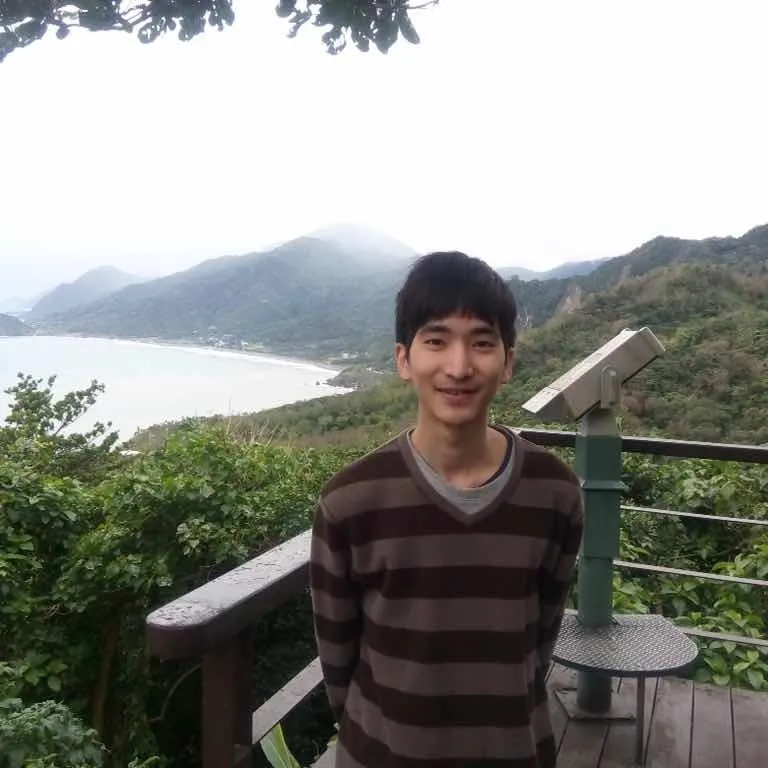
Francis is a research Lead at Labfront, responsible for data validation and analysis. He is interested in applying physics or math to medical research.
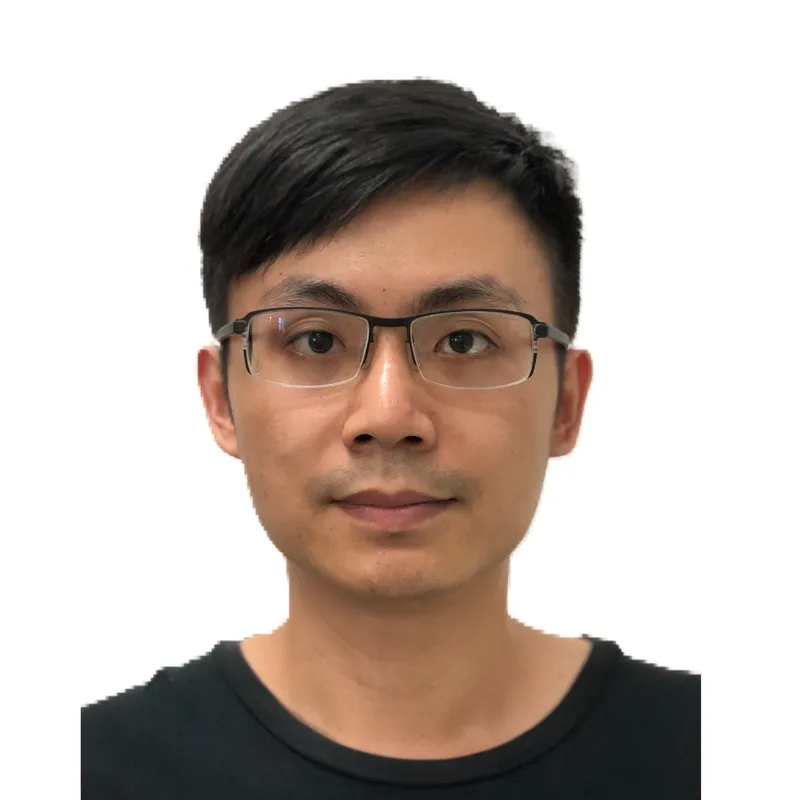
Han-Ping is the senior research lead (and chief plant caretaker) at Labfront, specializing in physiological data analysis. An alumnus of the BIDMC/Harvard's Center for Dynamical Biomarkers, Han-Ping uses his PhD in electrophysics to help Labfront customers convert raw physiological data into health insights. He does his best Python coding while powered by arm massages from his spiky-tongued cat, Pi.