Abstract
In the previous two posts, we examined the Motionlogger algorithm for deriving sleep/wake patterns and sleep parameters from raw acceleration (ACC) data. This post applied the same algorithms to the 6 nights of raw-ACC data obtained from Vivosmart 4 and compared the results with Motionlogger.
As described here, we found both the sleep/wake patterns and sleep parameters derived from Vivosmart 4 to be consistent with that of Motionlogger. The result suggests that an affordable, consumer wearable such as Vivosmart 4 can potentially reproduce the results from a more expensive, medical-grade actigraphic device like Motionlogger. Further validations for more data, especially for people with different sleep conditions, are still needed.
1. Comparison in Sleep/Wake Detection and Sleep Parameters
.png)
Figure 1 illustrates the sleep/wake patterns of the 6 nights derived from Motionlogger and Vivosmart 4 (the method was introduced in a previous post). The vertical dashed line in each subplot indicated the Motionlogger scored sleep onset.
Table 1 lists the consistent and inconsistent results between Motionlogger and Vivosmart 4. The number in each cell represents the total sleep/wake minutes contained within the time intervals between sleep onset and offset for the 6 nights. Treating the Motionlogger result as the gold standard, the sensitivity (to detect sleep), specificity, negative predictive value, and accuracy of Vivosmart 4 are near 0.99, 0.93, 0.77, and 0.99, respectively.
.png)
Table 2 compares the differences in sleep parameters (introduced in the previous post) between Motionlogger and Vivosmart 4. The two watches showed good consistency as the mean differences of most of the parameters are smaller than 5 mins.
.png)
2. Conclusion
We examined the full algorithm utilized in the Motionlogger to derive nighttime sleep/wake patterns and sleep parameters from the raw acceleration signal. Both the sleep/wake patterns and sleep parameters derived from Vivosmart 4 are consistent with that of Motionlogger. The result suggests that an affordable, consumer wearable such as Vivosmart 4 can potentially reproduce the results from a more expensive, medical-grade actigraphic device. Further validations for more data, especially for people with different sleep conditions, are still needed.
3. References
[1] D. Fekedulegn, M. E. Andrew, M. Shi, J. M. Violanti, S. Knox, and K. E. Innes, "Actigraphy-based assessment of sleep parameters," Annals of Work Exposures and Health, vol. 64, no. 4, pp. 350-367, 2020.
[2] Ambulatory Monitoring, Inc., “Motionlogger user guide”
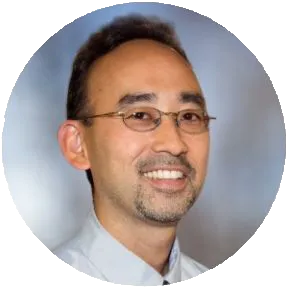
Dr. Ahn is an internal medicine physician with a background in physics/engineering and physiological signal analyses. He is the Chief Medical Officer at Labfront and an Assistant Professor in Medicine & Radiology at Harvard Medical School. Dr. Ahn is passionate about democratizing health sciences and exploring health from an anti-disciplinary perspective.
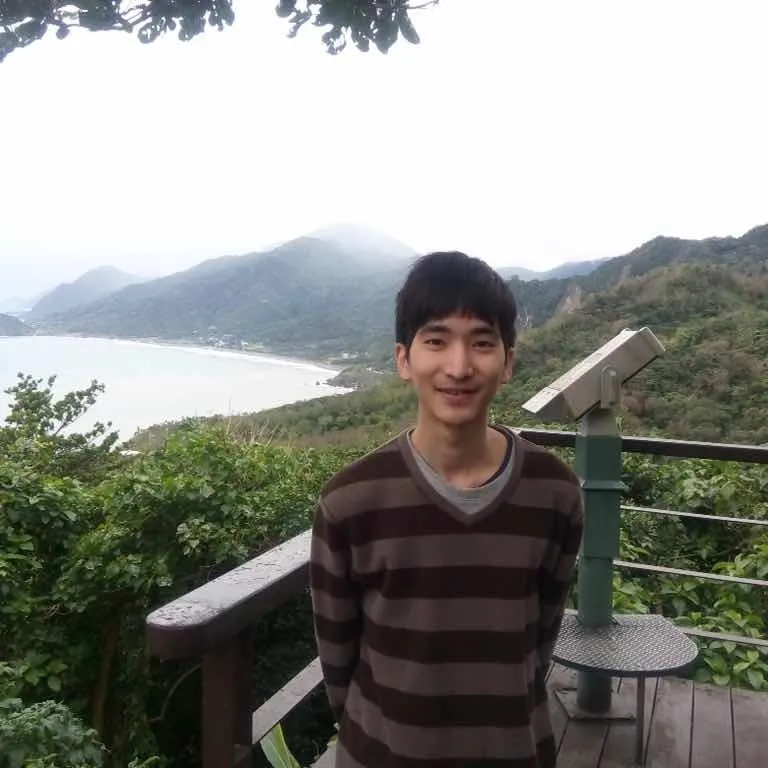
Francis is a research Lead at Labfront, responsible for data validation and analysis. He is interested in applying physics or math to medical research.
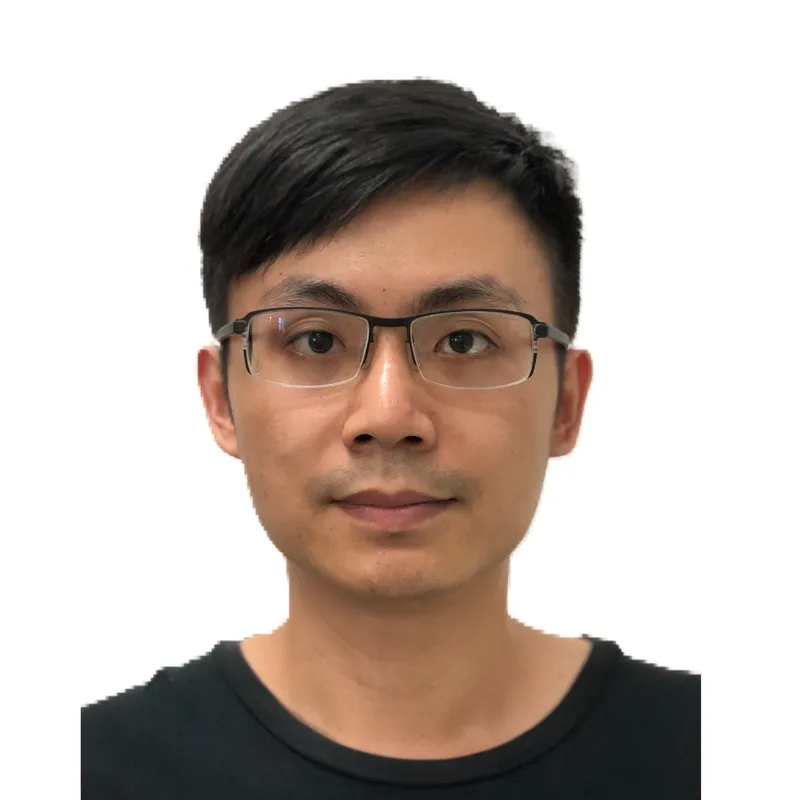
Han-Ping is the senior research lead (and chief plant caretaker) at Labfront, specializing in physiological data analysis. An alumnus of the BIDMC/Harvard's Center for Dynamical Biomarkers, Han-Ping uses his PhD in electrophysics to help Labfront customers convert raw physiological data into health insights. He does his best Python coding while powered by arm massages from his spiky-tongued cat, Pi.