Abstract
In our previous posts on sleep/wake detection using Actiwatch and Motionlogger, we demonstrated how two commonly used actigraphic devices, Motionlogger Sleep Watch (zero-crossing mode) and Actiwatch 2, derive nighttime sleep/wake patterns based on acceleration (ACC) signals. As can be seen, the processing methods for the two devices differ greatly with many contrasting, intermediate steps for adjusting various parameters. This post aims to compare the results derived from the two devices.
As described here, we found that data agreement between Motionlogger and Actiwatch 2 is low. Differences between them may arise from the parameter settings, including the choice of acceleration processing modes, the threshold for the noise of ACC signal, the threshold for sleep/wake detection, etc. The result implies that the parameters should be carefully determined, and are expected to be highly population-specific and personalized.
1. Comparison in Sleep/Wake Detection
Table 1 briefly reviews the sleep/wake detection methods for Motionlogger and Actiwatch 2 from the previous posts (Parts 2.1 & 3.1). In the whole process listed in Table 1, only the parameter of sleep/wake threshold (thsw) in Actiwatch 2 is tunable for users. Thus, we will compare the result derived from Motionlogger with Actiwatch 2 of various thsw.
%20(1).png)
Figure 1 compares the sleep/wake patterns of the 5 nights derived from Motionlogger with that from Actiwatch 2 under various thsw. Actiwatch 2 should be less sensitive in detecting awake with higher thsw values.
.png)
Treating the result from Motionlogger as the gold standard (for comparison purposes), the performance indices of Actiwatch 2 are listed in Tables 2 and 3. The overall sensitivity and accuracy of Actiwatch 2 are 0.94 and 0.93, respectively, which indicates that Actiwatch 2 performs well in detecting sleep. However, the overall specificity and negative predictive values are 0.41 and 0.31, respectively, which indicates that Actiwatch 2 and Motionlogger are not consistent in detecting awake times.
.png)
.png)
2. Discussion
We found that the consistency of sleep/wake detection between Motionlogger (zero-crossing mode) and Actiwatch 2 is low, which concurs with the conclusion of a previous study [1]. The differences between them may arise from the chosen parameter settings. The result might imply that one’s sleep habits or sleep conditions are not easily captured by a universal set of parameters. In other words, the parameter determinations are expected to be highly population-specific and personalized.
3. References
[1] L. J. Meltzer, C. M. Walsh, J. Traylor, and A. M. Westin, "Direct comparison of two new actigraphs and polysomnography in children and adolescents," Sleep, vol. 35, no. 1, pp. 159-166, 2012.
[2] Ambulatory Monitoring, Inc., “Motionlogger user guide”
[3] Respironics, “Instruction manual of Actiwatch Communication and Sleep Analysis Software.“
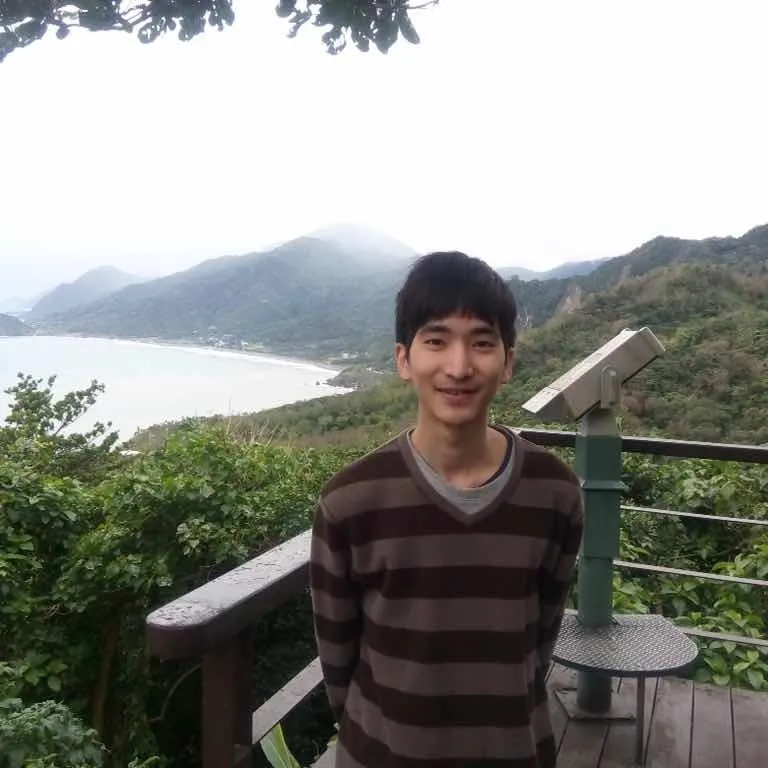
Francis is a research Lead at Labfront, responsible for data validation and analysis. He is interested in applying physics or math to medical research.
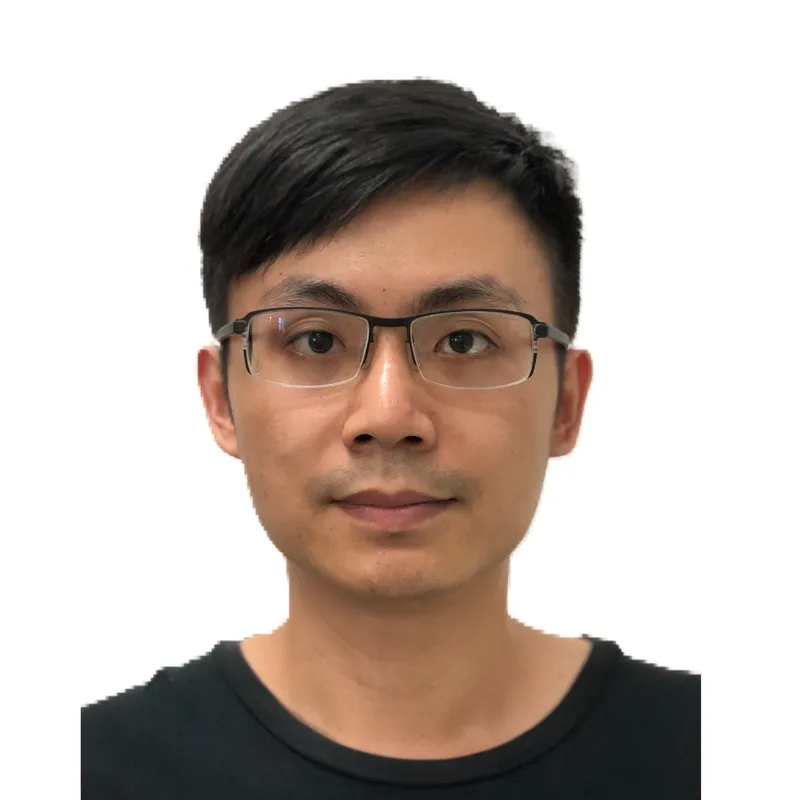
Han-Ping is the senior research lead (and chief plant caretaker) at Labfront, specializing in physiological data analysis. An alumnus of the BIDMC/Harvard's Center for Dynamical Biomarkers, Han-Ping uses his PhD in electrophysics to help Labfront customers convert raw physiological data into health insights. He does his best Python coding while powered by arm massages from his spiky-tongued cat, Pi.
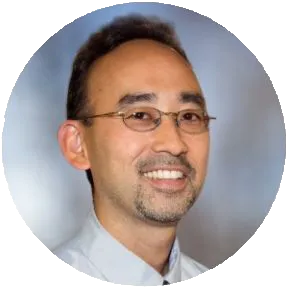
Dr. Ahn is an internal medicine physician with a background in physics/engineering and physiological signal analyses. He is the Chief Medical Officer at Labfront and an Assistant Professor in Medicine & Radiology at Harvard Medical School. Dr. Ahn is passionate about democratizing health sciences and exploring health from an anti-disciplinary perspective.