A Conversation with Dr. Han Ping Huang – Research Lead at Labfront
Can you introduce yourself and tell us a bit about how you ended up working at Labfront?
I’m Han Ping and I have been working at Labfront since February last year. I have my PhD in electro-physics which is very different from what I am doing now. Most people who study electro-physics go into semi-conductors and electronics however that never interested me. The last thing that I wanted was to end up an engineer in a big company and spend my days checking semi-conductor machines.
My passion is to combine different ideas together to make something new. While studying for my PhD I discovered the true power of interdisciplinary studies. This inspired me to create my own career path that fit my unique skillset as a data scientist.
Where did you first hear about data science as a career option and what drew you in?
The motivation was simple, for me data science is a very cozy and comfortable career path. In 2015, I had the chance to intern at one of the medical labs at Harvard and it was there that I discovered that this was the perfect career path for me. I use the word cozy to describe data science because I could do my analysis comfortably from the lab. Unlike my past work with lasers that involved complicated planning and preparations, this internship showed me that data analysis could be done remotely from a cozy office. The internship at Harvard was a beginning of sorts for me because it provided me with freedom and less stress as I explored the field of data science.

A lot of your work has involved physiological signal processing, can you explain what that is?
Human bodies vary a lot. Even when we look at the same types of signals like heart rate, there will always be quite a difference between you and me. It also depends on the device we use to collect the data because there are tons of things that can affect the data quality. Every researcher’s data will be different so we need to filter and process the information and derive the parameters we need. The data process is an important part of a data scientist’s job.
What are some ways you can compensate for those variations in data?
This is what I learnt while interning at Harvard, I learnt a decomposition method which is called empirical mode decomposition, EMD. Using that method, you can naturally do signal decomposition based on their natural properties to remove the natural noise from the signals.
We want clear documentation and verification posts to help keep Labfront fully transparent. It is through transparency that you build trust.
What sort of work do you do for Labfront as a Research Leader?
The first part of my role is dealing with the data and report generating. We discuss with the clients and understand what data they need for their research and then we generate the report they want.
The second part is helping the engineer team to check the data quality. Every time they upload a new version of the app or there is a new function installed, my team helps test it. We can check that the collected data quality is good enough for our analysis.
The last part of my role is documentation, we are building up Labfront’s resources. We want clients to easily understand what kinds of reports and data we can gather, the data analysis methods we use, and we want clear documentation and verification posts to help keep Labfront fully transparent. It is through transparency that you build trust.
Are those two Garmin watches I see on your wrist?
Yes! I am wearing two Garmin watches on my right wrist because I am now studying if there is a difference in the collected data quality if I wear the watches on the same wrist but in different positions. I hope in the future we can have a protocol that clients can refer to when setting up their research.
We want researchers to understand that Garmin devices are reliable for data collection even though they are small and widely used in the consumer market.
What are some challenges that researchers could run into when introducing wearables into their research and how is Labfront addressing those challenges?
If we focus on the participant part, the data collection method cannot be too annoying like needing to go to a lab or lying down completely still. This is why we chose the Garmin Vivosmart 4 smartwatches. These wearable devices are small and the battery can last up to one week.
We want researchers to understand that Garmin devices are reliable for data collection even though they are small and widely used in the consumer market. Because of this, we are currently doing lots of validation studies comparing how Garmin and EKG devices collect data.
What do you think the future of wearables looks like for researchers?
In the future, I think we won’t need wearable devices. There are already a lot of sensors in our living environment, we just need to apply them to research. Even now Wi-Fi can be used to detect your heart rate and breathing patterns because it’s a signal. When the signal reflects from our chest it can identify even minute fluctuations. If we can decode that data, we can get lots of information from our bodies. Because of this, I think that in the future we won’t need participants to wear anything.
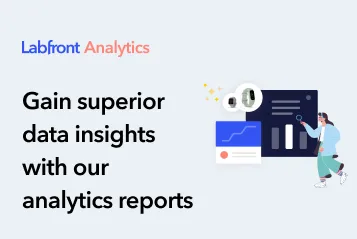
Save time on data processing and organization!
Know what you want but don’t want to code it yourself? Our analytics team helps researchers save time by distilling their data. All of our reports are backed by cited scientific research, so you can utilize the results with confidence.